Data Engineering Series – The Architecture
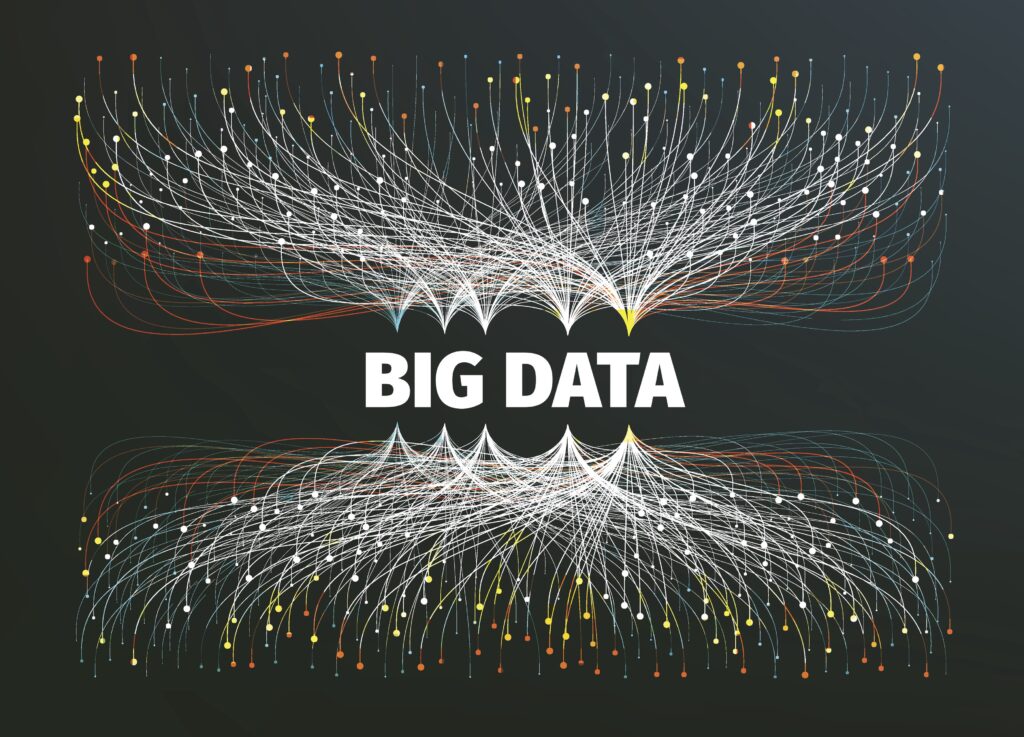
It is given that, Big data eco-system is the life blood of most organizations. But it is also true that there are a ton of organizations that face daunting challenges when it comes to handling Big Data correctly. Reflecting back on my experience over the years in fortune 500 companies including the most stringent of the environments where “data is the company” as well as having talked to many small and large companies in the industry, opportunity to improve the big data ecosystem are huge.
Do you find yourself asking the following questions…
- Technology – Engineering Challenges… Old arcane architecture (Hadoop?), running in your data center? Scaling, performance, maintainability, Data Quality, Data Governance and Observability issues? List of technology challenges is very long…
- People – Too many people doing the same thing and still cant get it right?
- Process – Manual processes and as a result delayed and inaccurate data? or communication break down between organizations such that the ownership of data is not clear?
Good news is Big Data, Machine Learning, Data Science ecosystem has evolved tremendously in recent years and there are tools that handle pretty much any problem in Data Engineering. But like they say… with power comes responsibility and with so many tools comes the complexity. If you misuse or pick the wrong tool for the job, you are in constant conundrum of data hell.
In this series of articles covering Data Engineering, I am planning on covering various technologies and components of a very generic data ecosystem architecture.
Without further ado… Fig. 1 covers a very high level end to end modern data ecosystem. I will cover more details and commentary on the components and technologies in more detail in coming articles.
Fig 1. Generic End to End Architecture for Data Ecosystem
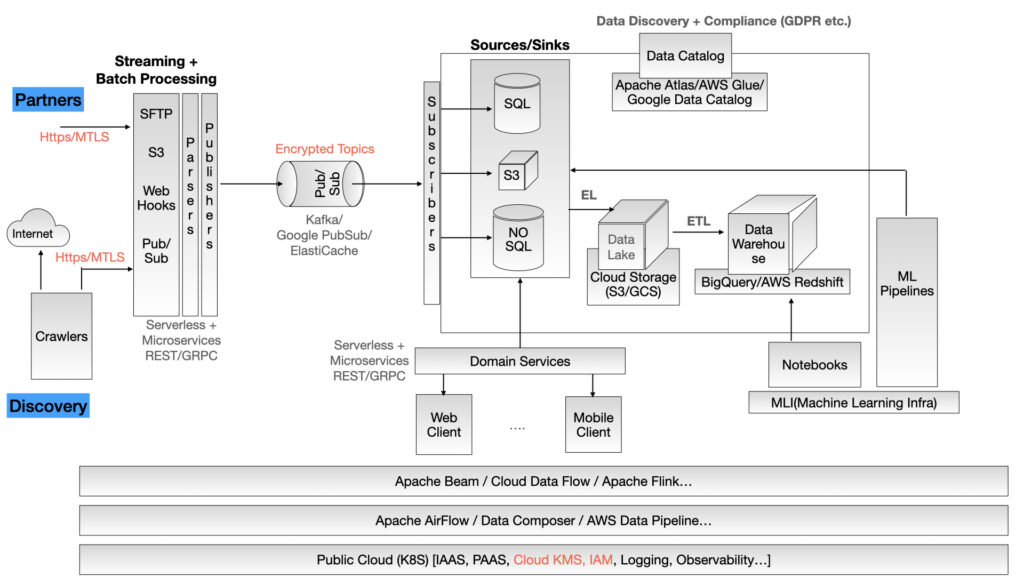
Always welcome thoughts.
Responses